Kweku A. Opoku-Agyemang
Working Paper Class 49
We propose a novel framework for economic analysis using Large World Models (LWMs)—spatially-aware, generative AI systems—to simulate realtime “what-if” scenarios with physical context. Unlike conventional models that abstract economic dynamics into equations, our framework leverages LWMs to simulate counterfactual scenarios with physical context, capturing the interplay of agents, infrastructure, and geography. The architecture combines (1) a transformer-based encoder to process multimodal data; (2) a recurrent simulator to model spatio-temporal evolution; and (3) a predictive engine to project outcomes of exogenous shocks. We demonstrate the model’s ability to reveal non-linear propagation effects—e.g., supply chain bottlenecks or regional spillovers— unobserved in equilibrium-based frameworks. Theoretical contributions include a formalization of spatial-economic feedback loops and a redefinition of economic dynamics as emergent properties of physical systems. Appendices provide regret bounds under varying assumptions, quantifying LWM performance. While empirical validation awaits richer data, simulations suggest LWMs outperform baseline models in capturing complexity, with implications for spatial economics and decision-making under uncertainty.
The views in this Working Paper Class are those of the authors, not necessarily of Machine Learning X Doing.
Opoku-Agyemang, Kweku A. (2025). "Large World Models for Economic Simulation: A Theoretical Framework." Machine Learning X Doing Working Paper Class 49. Machine Learning X Doing.
Copyright © 2025 Machine Learning X Doing Incorporated. All Rights Reserved.
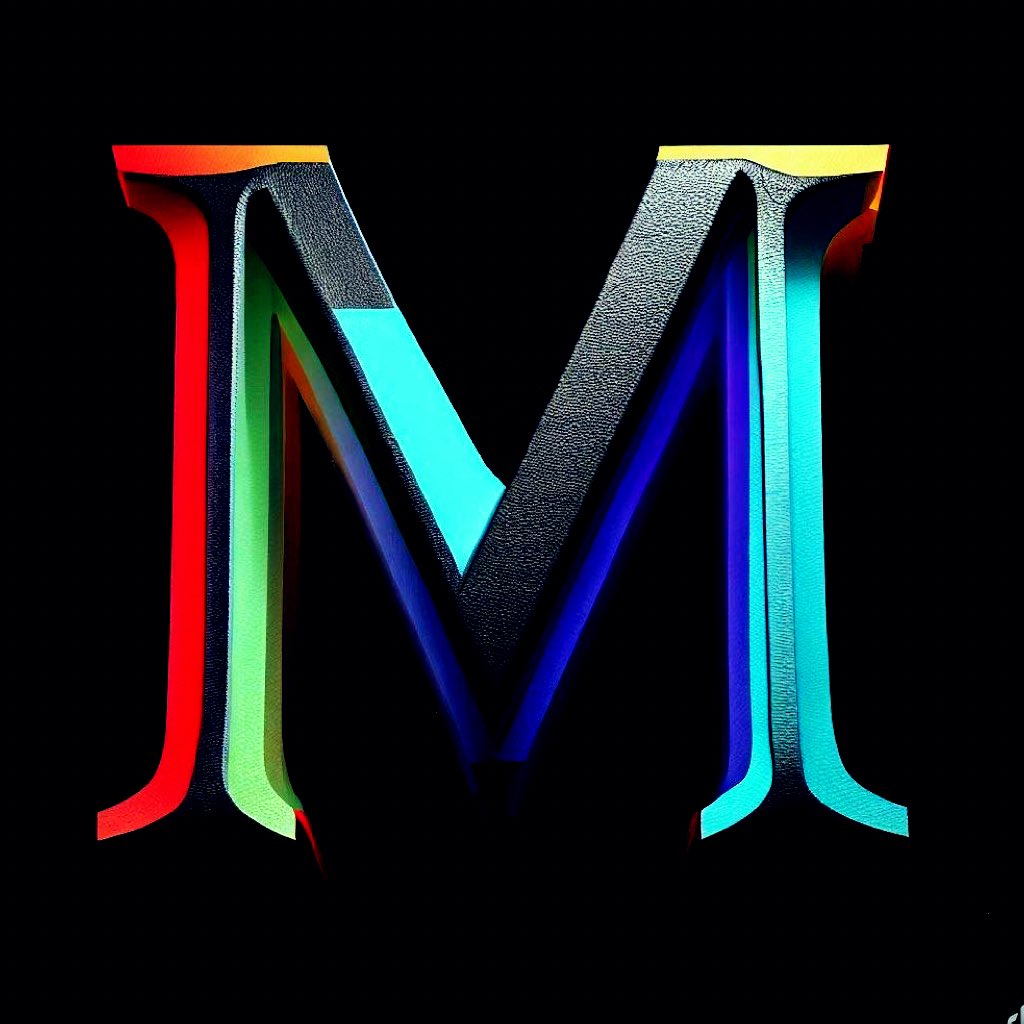