MACHINE LEARNING X DOING. May 15, 2025.
Introduction: Addressing a Critical Challenge in LLMs
Large Language Models (LLMs) are transforming industries, from customer support to decision-making, but they come with a significant challenge: Many business leaders worry about hallucinations—incorrect outputs that can cost thousands per incident. At Machine Learning X Doing, we’re tackling this problem head-on with a novel approach that combines biological interpretability with economic analysis. Our new paper, “Bio-Inspired Economics for Large Language Models: Optimizing Safety and Efficiency,” is now available as a working paper.
A Bio-Inspired Economic Framework: What We Did
Our framework draws inspiration from biological systems to view LLM circuits as agents in a marketplace, competing for computational resources. We propose a bio-inspired economic lens to:
- Analyze Costs and Benefits: Quantify the compute, environmental, and risk costs of LLM circuits, alongside benefits like accuracy and safety.
- Optimize with Graph Theory: Use graph-theoretic methods to scale circuit identification and optimization to thousands of circuits, reducing manual effort.
- Adapt Dynamically: Employ dynamic graph analysis to adjust circuit optimization across prompts, improving generalizability.
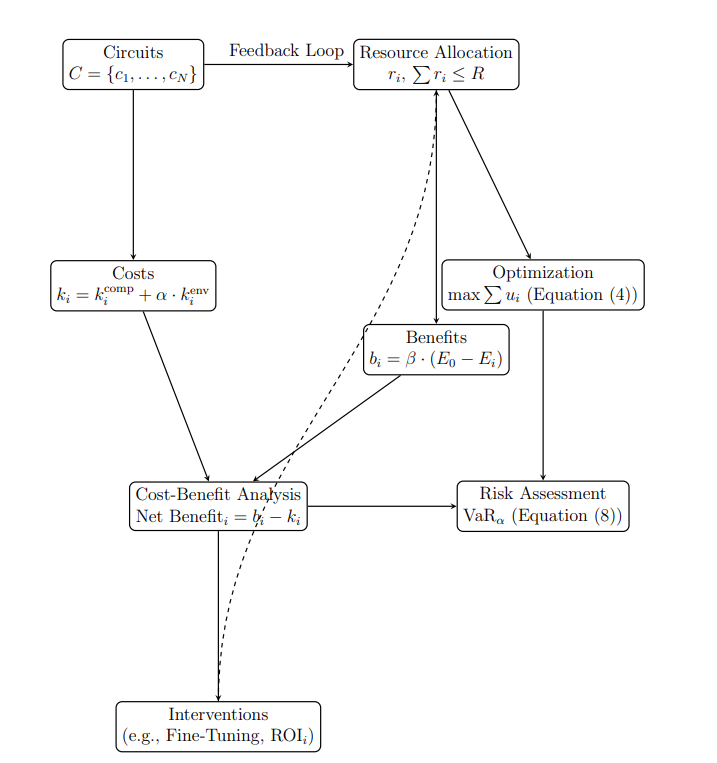
Figure 1: Our bio-inspired economic framework models LLM circuits as agents in a marketplace, optimizing resource allocation for safety and cost-effectiveness.
Key Results: A Hypothetical EcoNet-1B Case Study
In a hypothetical case study with EcoNet-1B (a 1-billion-parameter LLM), we fine-tuned a “factual recall” circuit, achieving:
- 15% Hallucination Reduction: From 20% to 17%, addressing a key concern for safe LLM deployment.
- $1.095 Million Annual Savings: In a customer support application, with a 600% ROI—demonstrating cost-effectiveness.
- Risk Mitigation: Reduced Value-at-Risk (VaR) from $22,400 to $19,000 daily, enhancing reliability.
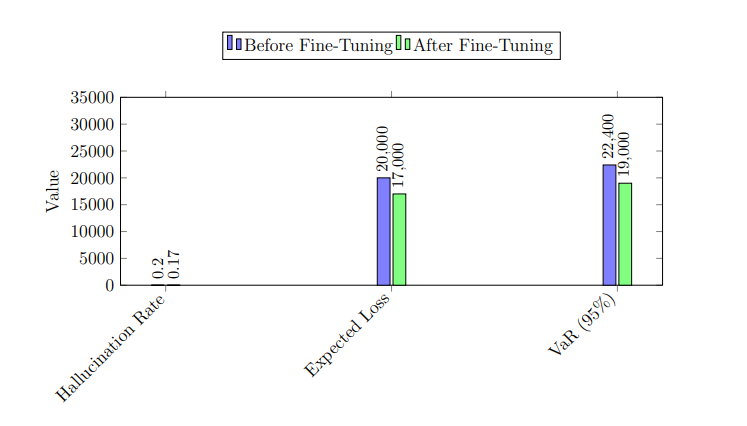
Figure 2: EcoNet-1B results show a 15% reduction in hallucinations and significant economic benefits, making LLMs safer and more efficient.
Why This Matters for AI Professionals and Consulting Firms
- For AI Professionals: Our framework enhances mechanistic interpretability, simplifying complex circuit analysis with economic metrics like ROI and VaR. The paper also offer scalable methods (graph theory, dynamic graph analysis) to handle real LLMs with thousands of circuits, addressing concerns about interpretability’s complexity.
- For Consulting Firms: As significant consulting buyers now demand AI integration, our framework provides a competitive edge. It enables you to offer clients safer, more cost-effective LLM solutions across industries like finance, healthcare, customer support and others. The focus on social impact aligns with public policy needs as well.
Read the Preprint and Let’s Collaborate.
We invite you to explore the full paper here. Interested in optimizing your LLM for safety, effectiveness and/or economic impact and more? We’re offering paid presentations and consulting engagements to help LLM/tech companies and consulting firms apply our framework. Send us a message; or email contact@machinelearningxdoing.com to schedule a session!
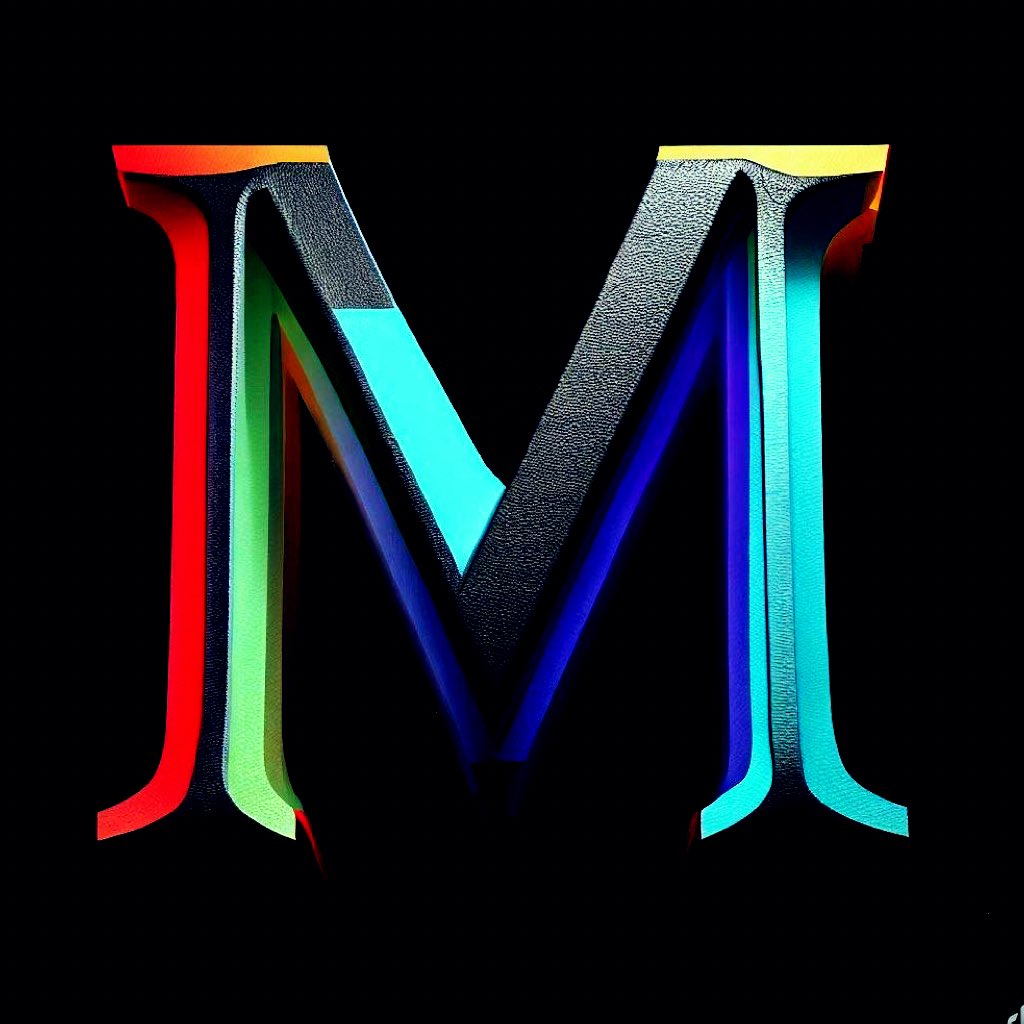
The views in this blog are those of the authors, not necessarily of Machine Learning X Doing. The technical paper is now available as a working paper.
Opoku-Agyemang, Kweku A. (2025). "Bio-Inspired Economics for Large Language Models: Optimizing Safety and Efficiency." Machine Learning X Doing Working Paper Class 54. Machine Learning X Doing.
Copyright © 2024 Machine Learning X Doing Incorporated. All Rights Reserved.