Kweku Opoku-Agyemang
Working Paper Class 54
Large Language Models (LLMs) have transformed natural language processing, yet their internal mechanisms remain opaque, posing challenges for interpretability and optimization. Recent work has applied biological analogies to dissect LLM reasoning through computational graphs, revealing how circuits contribute to behaviors like hallucinations. However, these insights lack a framework to evaluate their practical and societal implications. In this paper, we propose an economic lens to complement this biological approach, unpacking the economics of large language models in the process. We develop a novel methodology to analyze LLMs as economic systems, where circuits compete for computational resources (e.g., attention, activations) under constraints, akin to agents in a marketplace. To illustrate our approach, we present a case study with a hypothetical 1-billion-parameter LLM, EcoNet-1B, quantifying the costs and benefits of reasoning pathways identified in prior work. Using cost-benefit analysis and Value-at-Risk, we assess failure modes like hallucinations, showing that fine-tuning a “factual recall” circuit reduces hallucination rates by 15% (from 20% to 17%) at a compute cost of 5% of the original training ($500), yielding annual savings of $1.095 million in a customer support application. We further model the LLM as an ecosystem, where circuits “trade” influence to shape outputs, identifying “market failures” that lead to errors and offering economically grounded strategies to mitigate them. By bridging biology and economics, this work provides a unified bioeconomic framework to prioritize interpretability efforts, reduce societal risks, and guide sustainable LLM development. Our approach not only enhances the mechanistic understanding of LLMs but also makes their internal dynamics more actionable for engineers and policymakers, fostering broader engagement with interpretability research.
The views in this Working Paper Class are those of the authors, not necessarily of Machine Learning X Doing.
Opoku-Agyemang, Kweku A. (2025). "Bio-Inspired Economics for Large Language Models: Optimizing Safety and Efficiency." Machine Learning X Doing Working Paper Class 54. Machine Learning X Doing.
Copyright © 2025 Machine Learning X Doing Incorporated. All Rights Reserved.
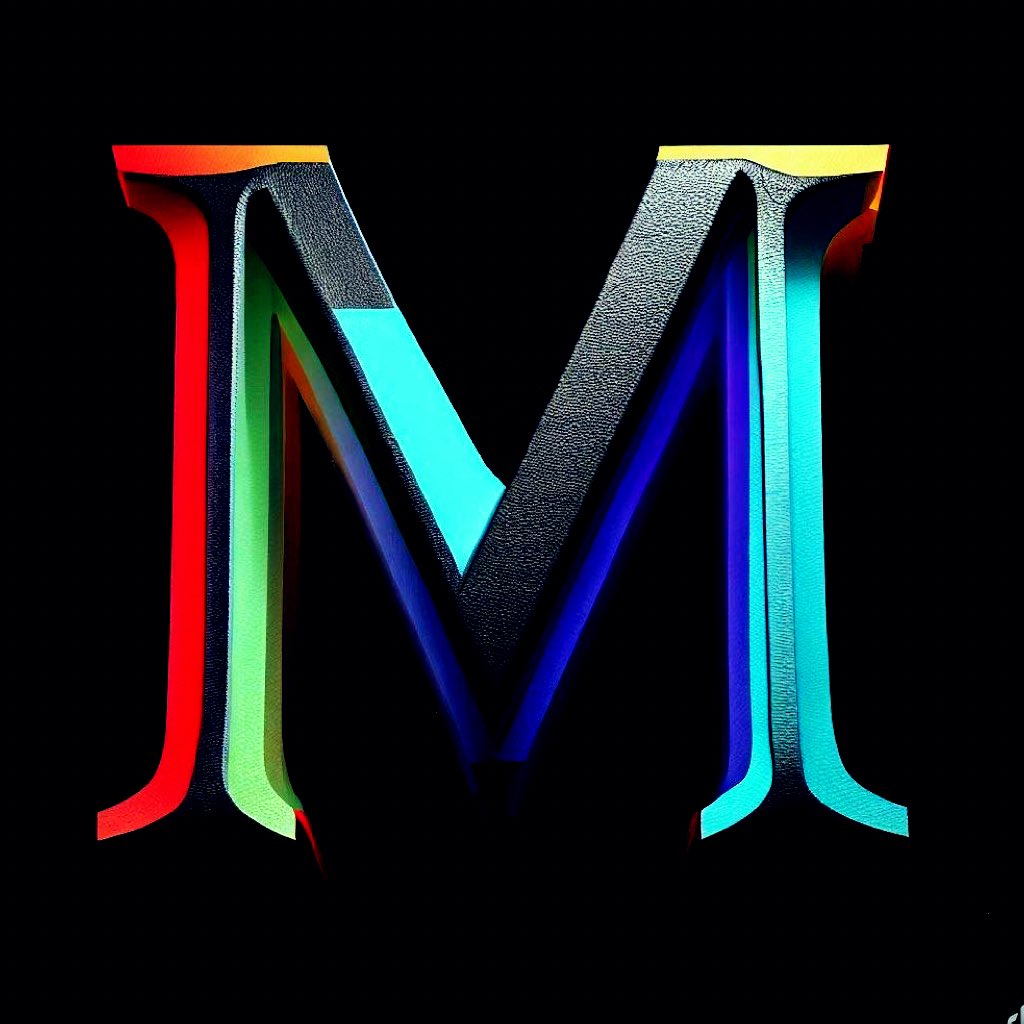