Kweku Opoku-Agyemang
Working Paper Class 31
We propose a novel framework for causal inference and natural experiment detection in self-driving cars. We model the car’s environment as a dynamic causal graph, where the nodes represent variables that affect the car’s performance, and the edges represent causal relationships that are updated in real-time based on the data collected by the car’s sensors and cameras. We use a counterfactual engine to generate hypothetical scenarios that could have happened if the car had taken a different action or faced a different situation, and compare them with the actual outcomes. We use a natural experiment detector to identify situations where the car is exposed to a natural or quasi-experimental variation that affects one or more variables in the causal graph, and use them to estimate the causal effects of interest. We show that our framework can learn new causal relationships, test and refine causal hypotheses and assumptions, and evaluate and optimize the car’s decision-making and performance. We also provide feedback and explanations to the human driver or passengers if needed. We derive regret bounds for our framework that depend on the number of natural experiments encountered, the quality of the counterfactual engine, and the complexity of the causal graph. We also discuss regret bounds for learning and updating the causal graph from data and feedback using online learning algorithms; for identifying situations where natural experiments occur using anomaly detection methods that can detect changes and outliers in data; and for evaluating and optimizing the quality and validity of natural experiments using evaluation methods that can account for selection bias, endogeneity, spillover effects, compliance issues, and measurement error.
The views in this Working Paper Class are those of the authors, not necessarily of Machine Learning X Doing.
Opoku-Agyemang, Kweku A. (2023). "Causal Inference and Natural Experiment Detection in Self-Driving Cars: A Dynamic Causal Graph Approach." Machine Learning X Doing Working Paper Class 31. Machine Learning X Doing.
Copyright © 2023 Machine Learning X Doing Incorporated. All Rights Reserved.
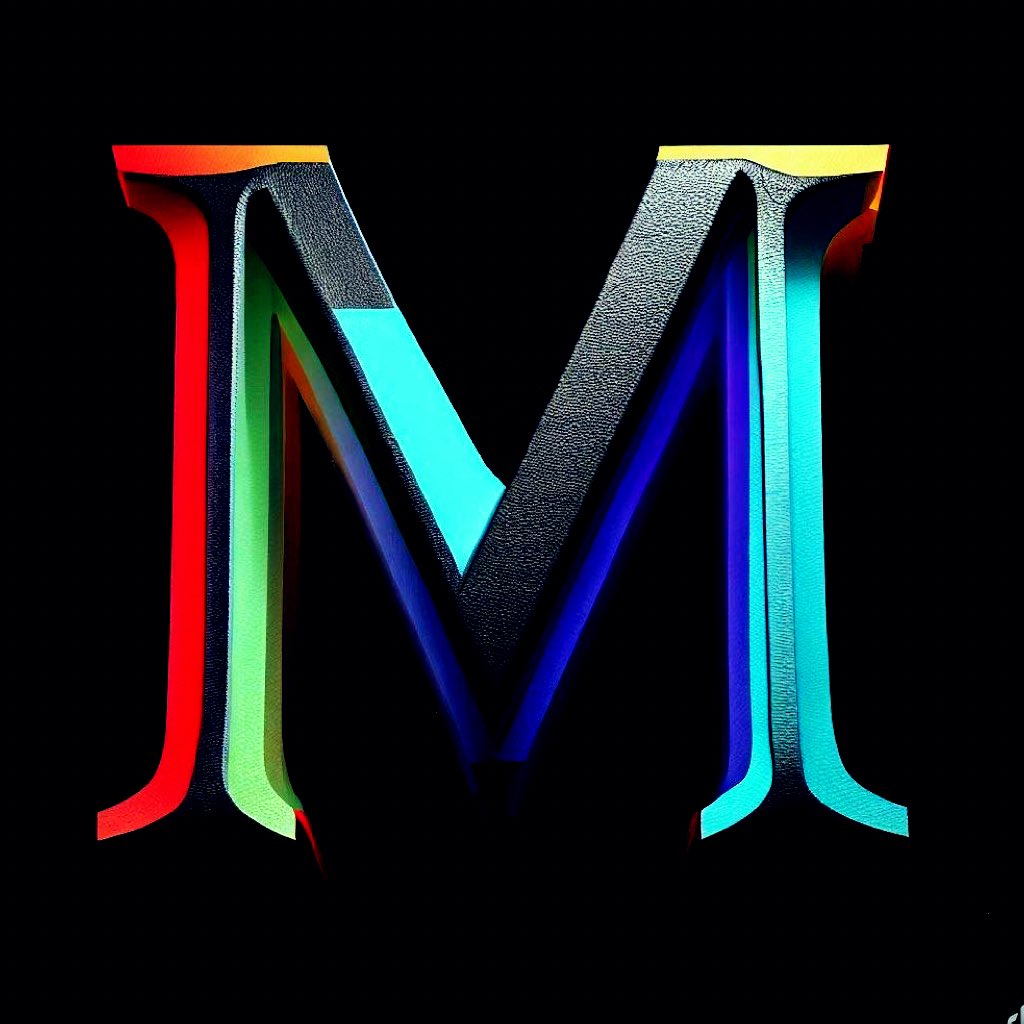