Kweku Opoku-Agyemang
Working Paper Class 53
We propose Causal Inferencing, a novel framework that integrates causal inference into the real-time inference pipeline of general-purpose AI systems, such as large language models. By modeling Causal Inferencing as a sequential decision-making problem, we enable dynamic construction and querying of structural causal models (SCMs) to produce causally-informed responses. We establish theoretical guarantees, including consistency of causal effect estimation under identifiable SCMs, a sublinear regret bound for sequential predictions, and polynomial-time complexity for sparse graphs. Under stronger assumptions, we derive a tighter regret bound, reducing dependence on the hypothesis space size. These results are extended through scalable enhancements, including approximate causal discovery and hierarchical hypothesis spaces, addressing large-scale problems. Causal Inferencing bridges statistical causal inference and AI, offering applications in econometrics, healthcare, and interactive AI interfaces, with robust guarantees for interpretability and performance.
The views in this Working Paper Class are those of the authors, not necessarily of Machine Learning X Doing.
Opoku-Agyemang, Kweku A. (2025). "Causal Inferencing: Real-Time Causal Reasoning in AI Systems." Machine Learning X Doing Working Paper Class 53. Machine Learning X Doing.
Copyright © 2025 Machine Learning X Doing Incorporated. All Rights Reserved.
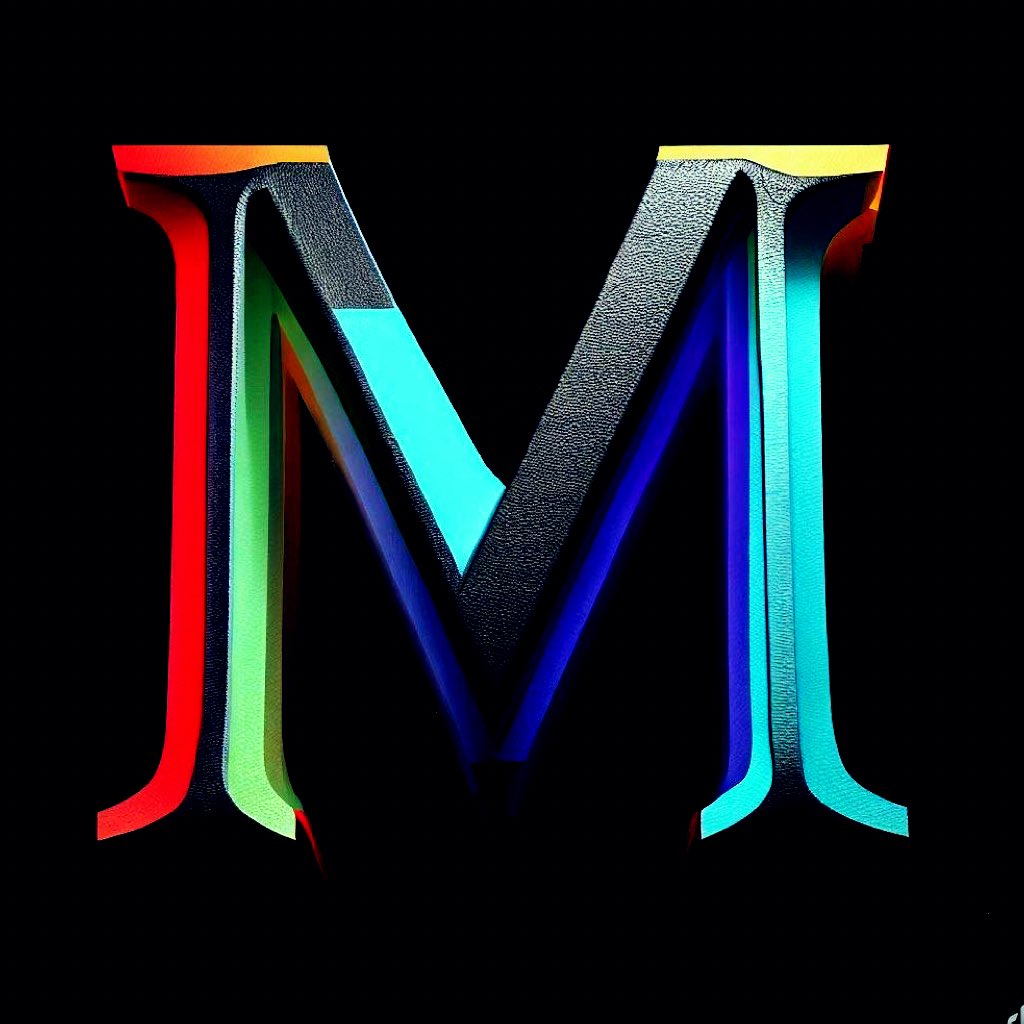