Kweku A. Opoku-Agyemang
Working Paper Class 26
This paper develops a framework for auction design for AI chatbots, which are conversational agents that use natural language to interact with users. We apply deep reinforcement learning (DRL) to optimize the bidding strategies of the advertisers, who compete for ad slots in the chatbot conversations. We also consider the goals and constraints of the chatbot owner or developer, who acts as the seller in our setting. We use transformer-based language models (TLMs) to analyze the conversational data of the users, who are the potential buyers of the advertised products or services. We extend Border (1991), which shows how to construct an auction from a given reduced form, which is a function that maps each bidder’s type to his probability of winning. We show how to use DRL and TLMs to learn and generate more realistic and flexible reduced forms, which can capture the complex preferences and behaviors of advertisers, and users, as well as the features and contexts of the ad slots and the chatbot conversations. We also show how to use market design, mechanism design and user interface design principles to create and improve relevant ad markets and institutions. We conclude with some guidelines and best practices for auction design for AI chatbots.
The views in this Working Paper Class are those of the authors, not necessarily of Machine Learning X Doing.
Opoku-Agyemang, Kweku A. (2023). "Chatbot Auctions: How to Use Deep Reinforcement Learning and Transformer-Based Language Models to Create and Improve Advertising Markets and Institutions." Machine Learning X Doing Working Paper Class 25. Machine Learning X Doing.
Copyright © 2023 Machine Learning X Doing Incorporated. All Rights Reserved.
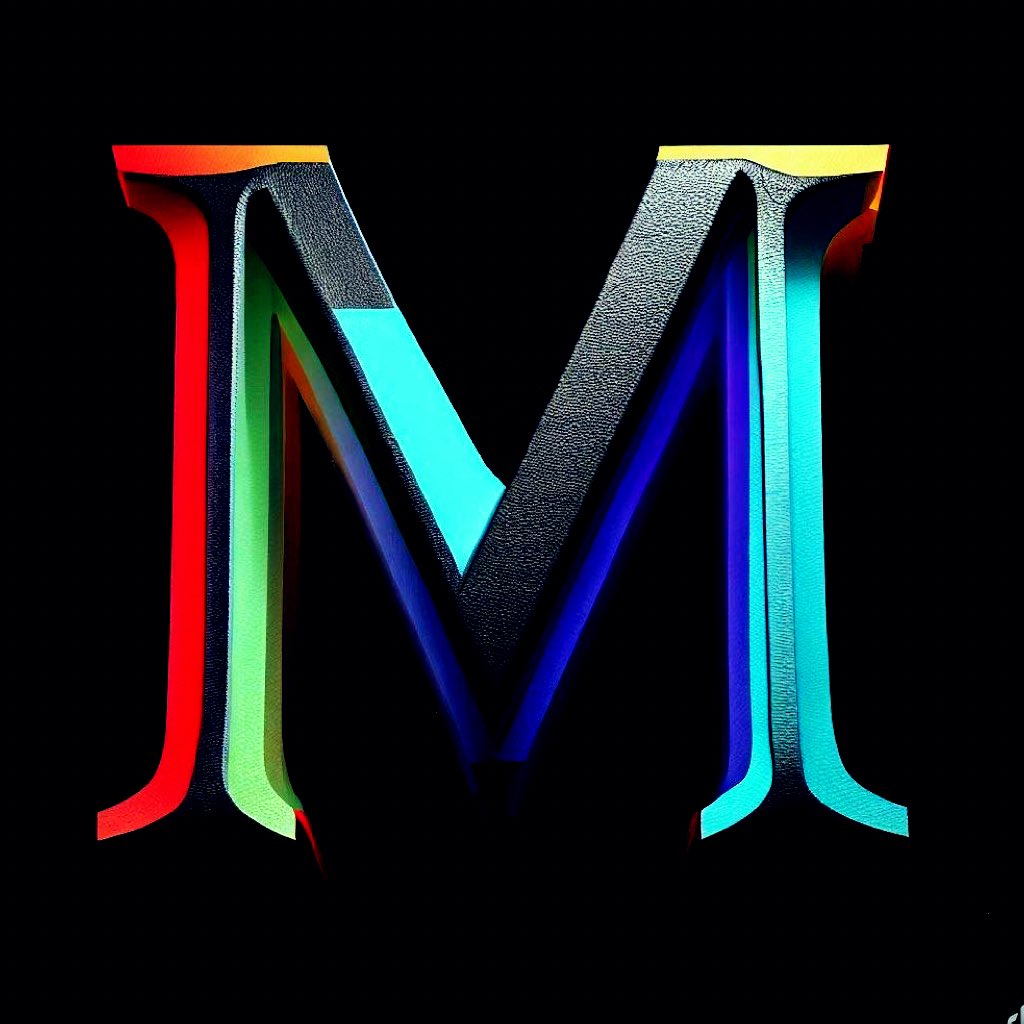