Kweku Opoku-Agyemang
Working Paper Class 36
Homotopy type theory is a newer branch of mathematics that combines ideas from topology, logic and category theory. It aims to provide a unified foundation for mathematics based on the notion of homotopy, which is a way of comparing shapes by bending and stretching them without tearing or gluing. Homotopy type theory also introduces a new concept of equality, called homotopy equivalence, that captures the idea of being the same up to deformation. This allows for more flexible and expressive reasoning about mathematical objects and structures. In this paper, we explore how homotopy type theory can be useful for developing new ways of representing and manipulating data, as well as for designing more robust and adaptable algorithms and efficient learning architectures. We show how homotopy type theory can enable novel forms of data abstraction, transformation and integration, as well as new methods of inference, optimization and generalization. We also discuss how homotopy type theory can help us model and understand the learning processes of animals and humans, who can learn from very small amounts of data and adapt to changing environments. We argue that homotopy type theory can offer a new perspective and a new toolkit for advancing the field of machine learning. In a brief appendix, we discuss how to implement and evaluate homotopy type theory based machine learning models and systems, and compare them with the existing ones, such as Q-transformers. We also briefly explain why homotopy type theory related machine learning models may have some advantages over Q-transformers in terms of scalability, robustness, and interpretability.
The views in this Working Paper Class are those of the authors, not necessarily of Machine Learning X Doing.
Opoku-Agyemang, Kweku A. (2024). "Data architectures and machine learning for efficiently modeling the learning processes of humans and animals." Machine Learning X Doing Working Paper Class 36. Machine Learning X Doing.
Copyright © 2024 Machine Learning X Doing Incorporated. All Rights Reserved.
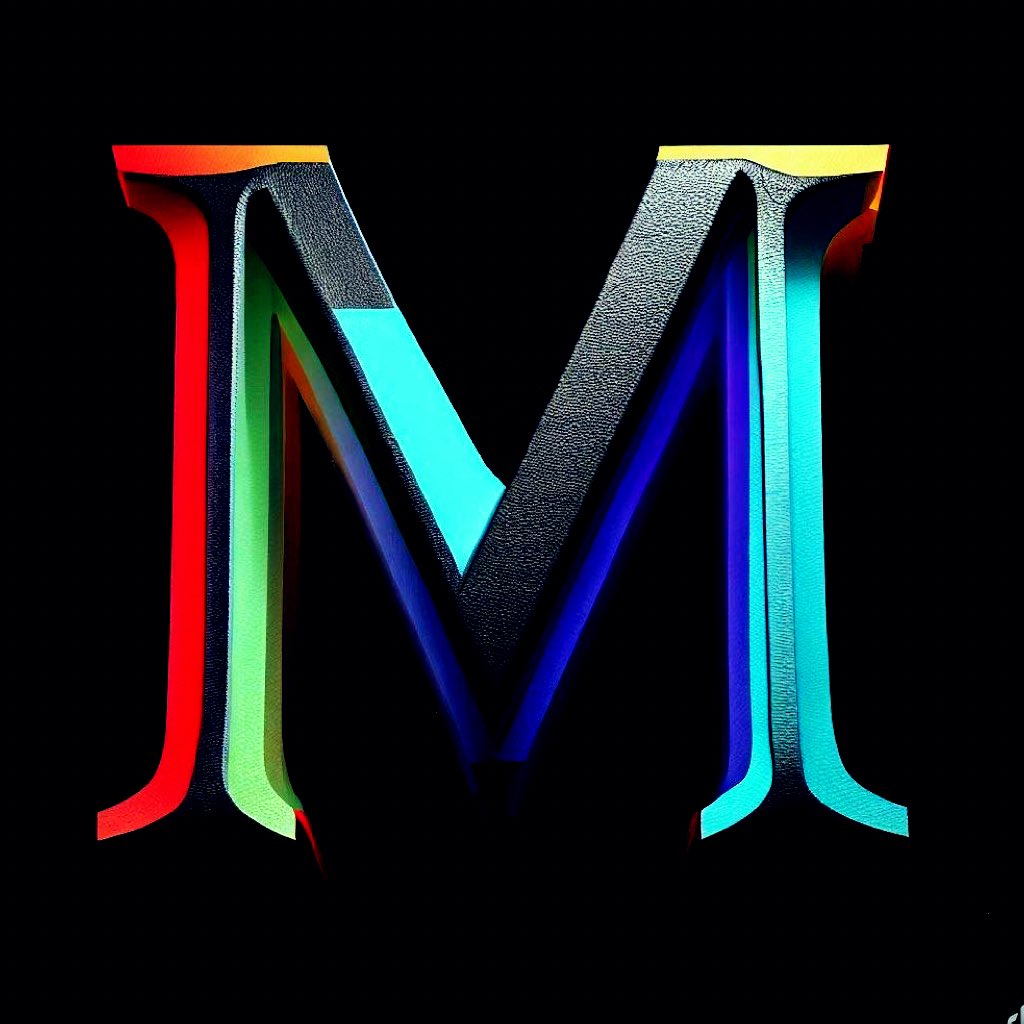