Kweku A. Opoku-Agyemang
Working Paper Class 7
In this note, we present a non-parametric causal transformer for estimating heterogeneous treatment effects. In the potential outcomes framework with unconfoundedness, we show that causal transformers are pointwise consistent for the true treatment effect and have an asymptotically Gaussian and centered sampling distribution. We also discuss a practical method for constructing asymptotic confidence intervals for the true treatment effect that are centered at the causal transformer estimates. Our theoretical results rely on a generic Gaussian theory for a large family of transformer models. To our knowledge, this is the first set of results that allows any type of transformer, including encoder-decoder and encoder-only models, to be used for provably valid statistical inference in the econometric sense.
The views in this Working Paper Class are those of the authors, not necessarily of Machine Learning X Doing.
Opoku-Agyemang, Kweku A. (2023). "Generalized Transformers." Machine Learning X Doing Working Paper Class 7. Machine Learning X Doing.
Copyright © 2023 Machine Learning X Doing Incorporated. All Rights Reserved.
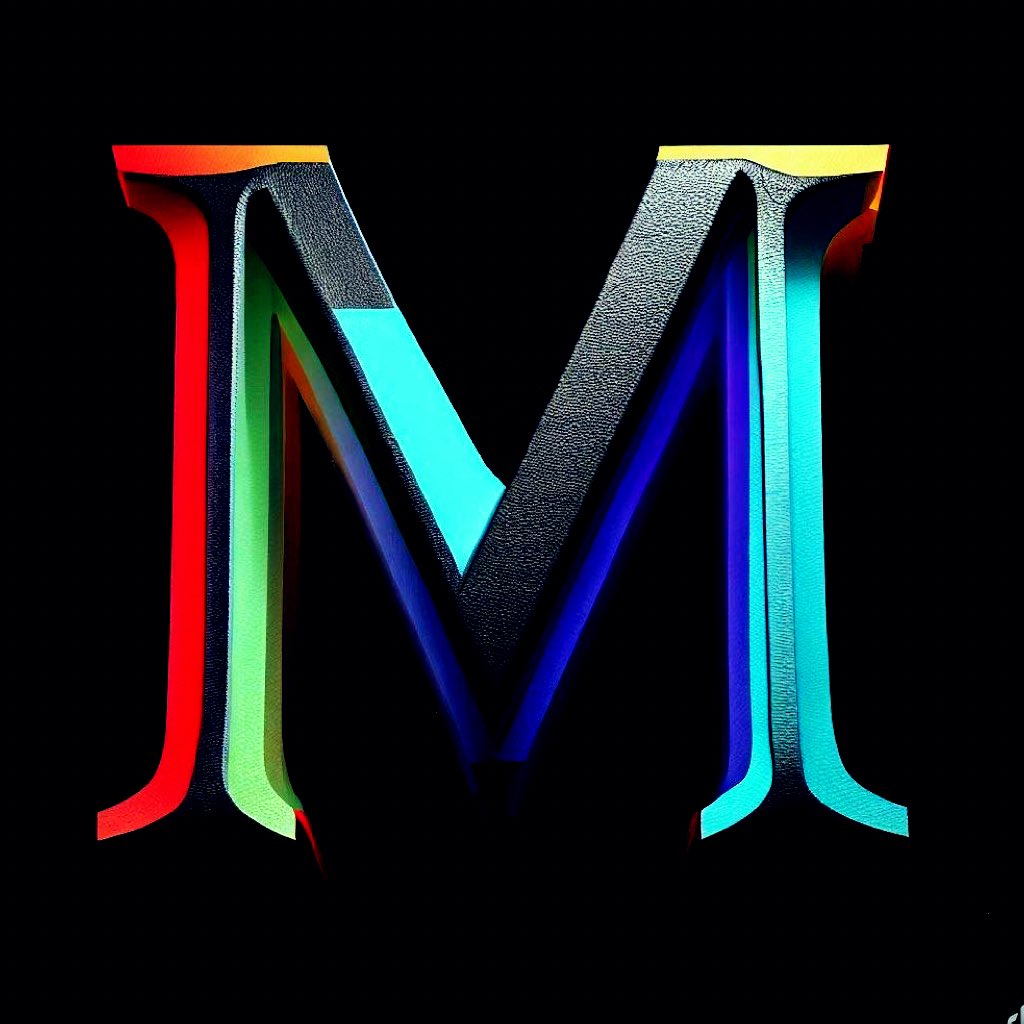