Kweku A. Opoku-Agyemang
Working Paper Class 1
In livestreams and other real-time data applications of linear contextual bandits, the treatment assignment may depend on some observable characteristic of the context, such as a threshold or a cutoff. This creates unique challenges for estimating the causal effects of the actions, as well as for balancing exploration and exploitation. We propose a novel approach that leverages the regression discontinuity design (RDD) framework for linear contextual bandits. We develop two algorithms, RDD’s BLTS and RDD’s BLUCB, that use RDD-based estimation and exploration methods in the IPTW context of Dimakopoulou, Zhou, Athey and Imbens (2017). Specifically, RDD’s BLTS uses Bayesian linear regression with balancing weights to estimate the potential outcomes and select the actions with the highest posterior mean. RDD’s BLUCB uses local linear regression with upper confidence bounds to estimate the potential outcomes and select the actions with the highest upper bound. We provide theoretical guarantees on the regret bounds of our algorithms, which depend on the distance from the cutoff and the bandwidth of the RDD. We assume that the potential outcomes and the covariates are continuous and smooth in the forcing variable that determines the treatment assignment, and that the optimal bandwidth for each context is chosen to minimize some criterion such as mean squared error or coverage error probability.
The views in this Working Paper Class are those of the authors, not necessarily of Machine Learning X Doing.
Opoku-Agyemang, Kweku A. (2023). "Livestream: Contextual Bandits meet Regression Discontinuity Designs." Machine Learning X Doing Working Paper Class 1. Machine Learning X Doing.
Copyright © 2023 Machine Learning X Doing Incorporated. All Rights Reserved.
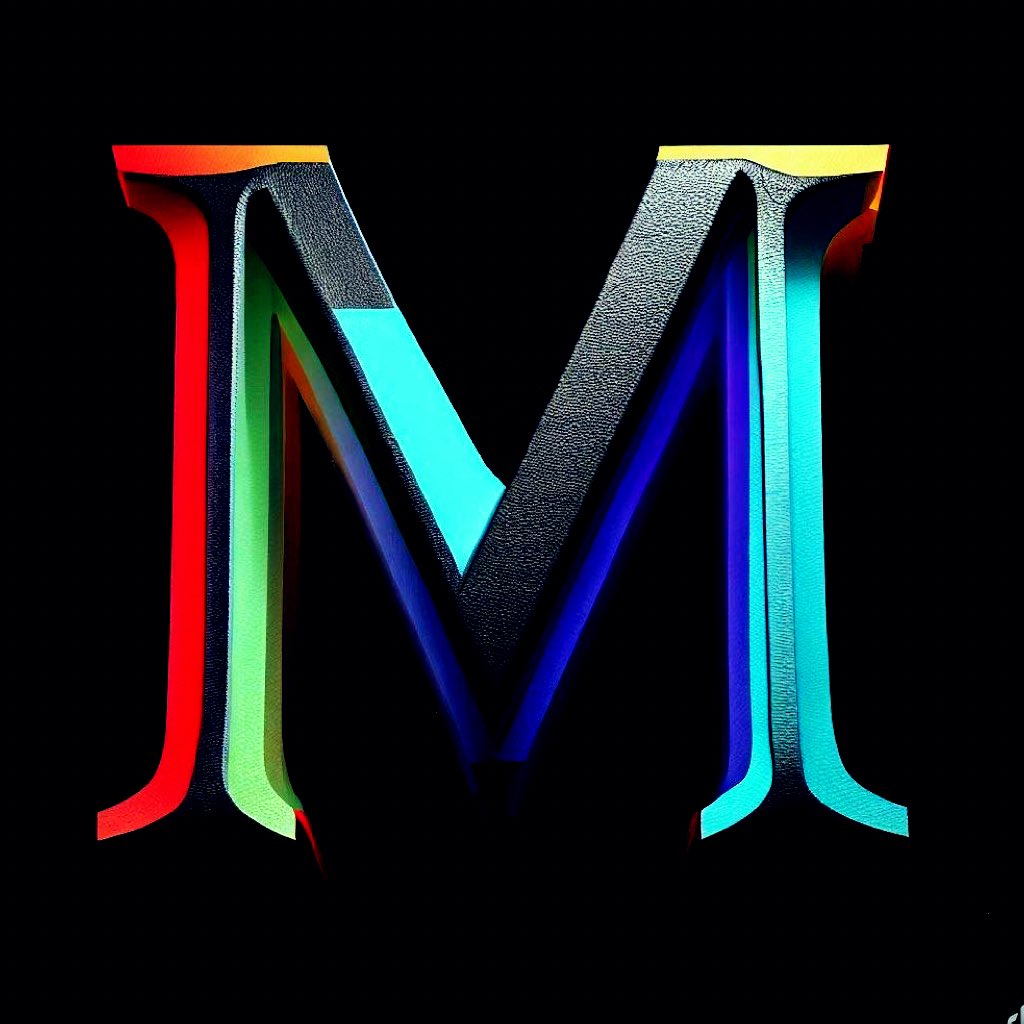