Kweku Opoku-Agyemang
Working Paper Class 42
This paper generalizes the classic Stiglitz-Weiss model using measure theory and integration to better analyze creditworthiness under information asymmetries. We revisit the original model, address its limitations, and introduce a measure-theoretic framework to handle the distribution of borrower types and associated risks. Our model accounts for a broad spectrum of borrower behaviors, including those underrepresented in traditional models, and derives new equilibrium conditions and credit rationing outcomes. We introduce a novel information asymmetry, termed information dispersion, where gaining information about one dimension of a borrower’s type increases uncertainty about other dimensions. This arises from the multidimensional nature of borrower types and the dynamic information structure in informal markets. Simulations illustrate this concept. Our findings offer a rigorous approach to understanding credit markets under information asymmetries, potentially improving credit access and financial stability.
The views in this Working Paper Class are those of the authors, not necessarily of Machine Learning X Doing.
Opoku-Agyemang, Kweku A. (2024). "Measuring Up to Stiglitz-Weiss: Measure Theory, Credit and Information Dispersion." Machine Learning X Doing Working Paper Class 42. Machine Learning X Doing.
Copyright © 2024 Machine Learning X Doing Incorporated. All Rights Reserved.
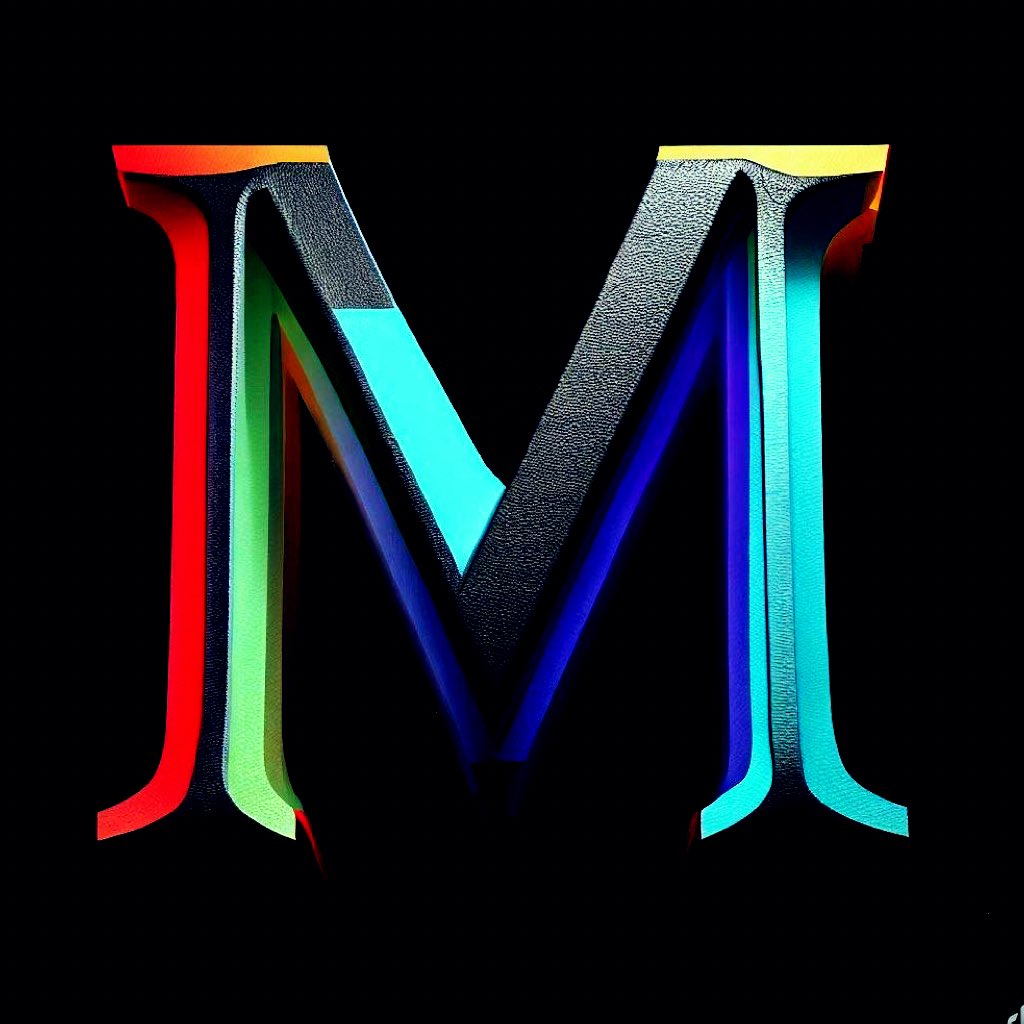