Kweku Opoku-Agyemang
Working Paper Class 14
This paper focuses on how human feedback can improve an randomized controlled trial intervention that affects people’s behavior or outcomes. For example, the intervention could be a personalized message that encourages people to exercise more or eat healthier. Human feedback could be ratings, preferences, emotions, or rewards that people give after receiving the intervention. We propose a two-stage method: First, we run randomized controlled trials (RCTs) to compare different kinds of human feedback or different ways to calculate rewards for the same intervention. Second, we use reinforcement learning from human feedback (RLHF) to optimize the intervention based on the best kind of feedback or reward. The quality of our method depends on several factors, such as: how many kinds of feedback or rewards we compare in the first stage; how easy or hard it is to measure each kind of feedback or reward; how consistent or variable each kind of feedback or reward is; how long we run the second stage and how much we care about future outcomes; and how well our RLHF algorithm can learn from human feedback and adapt to new situations. Our goal is to find a balance between these factors that leads to the most effective intervention. We close with policy implications.
The views in this Working Paper Class are those of the authors, not necessarily of Machine Learning X Doing.
Opoku-Agyemang, Kweku A. (2023). "Reinforcement Learning from Human Feedback via Randomized Experiments." Machine Learning X Doing Working Paper Class 14. Machine Learning X Doing.
Copyright © 2023 Machine Learning X Doing Incorporated. All Rights Reserved.
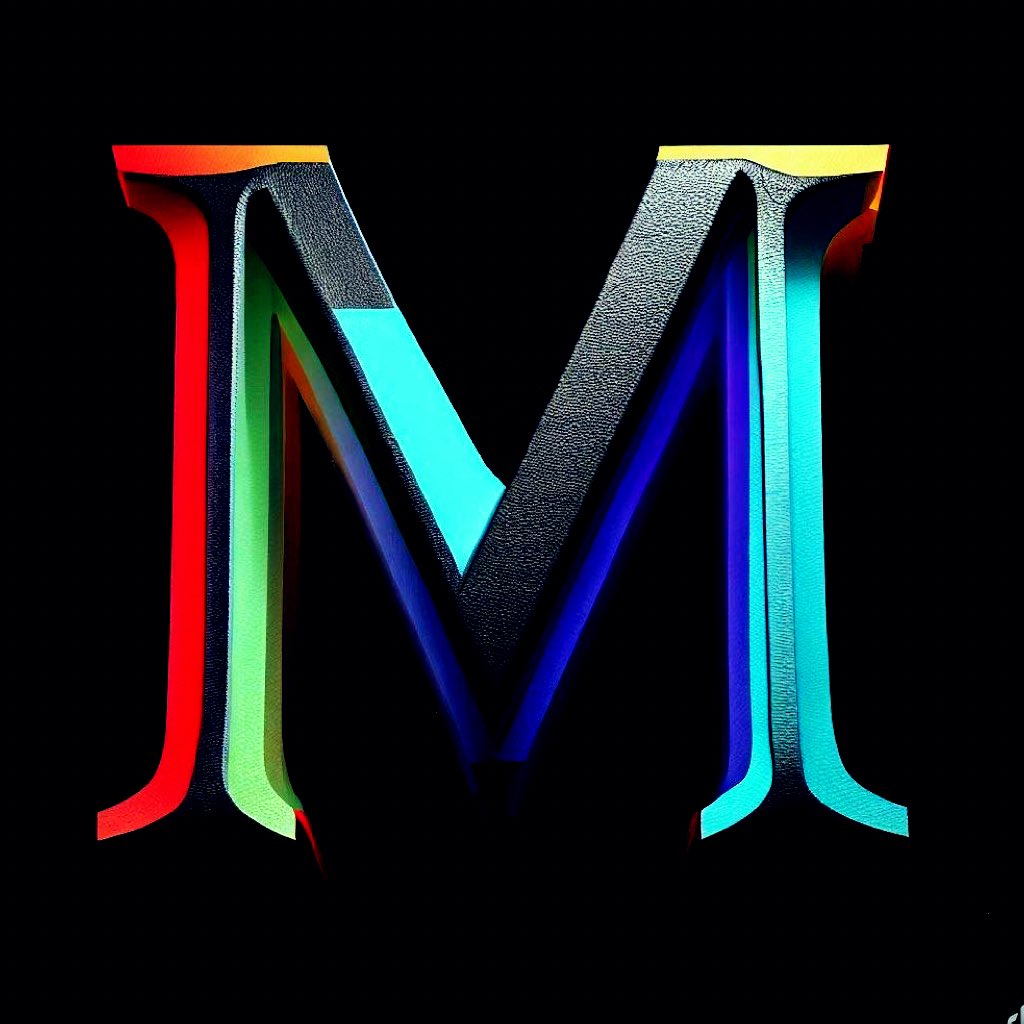