Kweku A. Opoku-Agyemang
Working Paper Class 25
In this paper, we explore the connections between subspace designs and directed acyclic graphs (DAGs), and how they can enhance each other. Subspace designs are mathematical objects that involve arranging subspaces of a finite vector space into sets that satisfy certain combinatorial properties. DAGs are tools for representing the probabilistic relationships among a set of variables. We show that subspace designs can improve DAGs in two ways: by providing efficient methods for encoding and decoding information in high-dimensional spaces, and by discovering new patterns and structures in data. We use subspace designs to construct error-correcting codes, which can help to recover the original information from noisy or corrupted messages that are passed among nodes in the DAG using inference algorithms. We also use subspace designs to construct dimension expanders, which can help to find low-dimensional embeddings of high-dimensional data that reveal hidden features or clusters. We close with subspace design applications to causal diagrams, regression, clustering and classification.
The views in this Working Paper Class are those of the authors, not necessarily of Machine Learning X Doing.
Opoku-Agyemang, Kweku A. (2023). "Subspace Designs and Directed Acyclic Graphs: An Approach to High-Dimensional Causality." Machine Learning X Doing Working Paper Class 25. Machine Learning X Doing.
Copyright © 2023 Machine Learning X Doing Incorporated. All Rights Reserved.
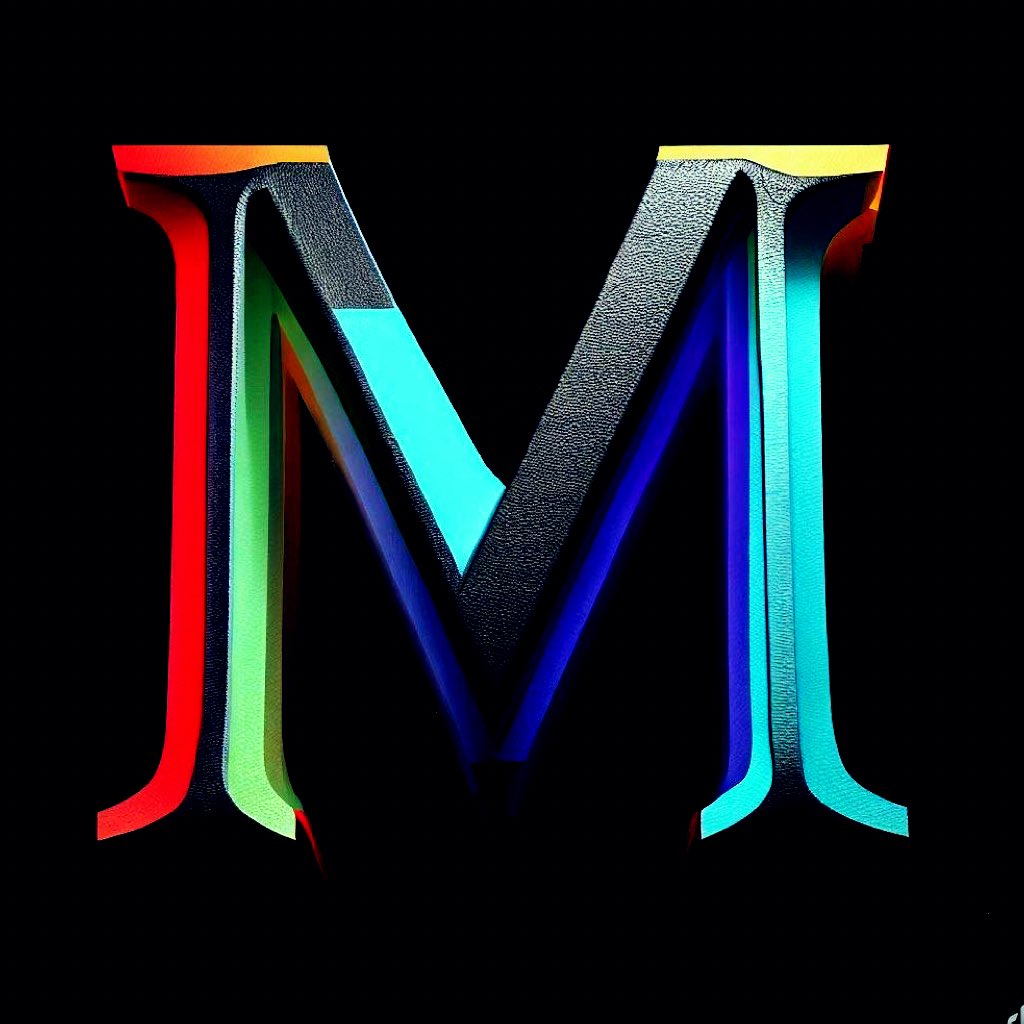