Kweku A. Opoku-Agyemang
Working Paper Class 6
To limit noncompliance and attrition issues, this paper introduces a treatment dummy variable concept for data contexts where the treatment status of an individual is not fully observed or determined by the researcher, but depends on how much the instrument affects the probability of receiving the treatment. I first show that, compared to a standard dummy variable, these special dummy variables improve the precision and efficiency of estimating the Complier-Average Causal Effect (CACE). In such cases, using a standard dummy variable to indicate the treatment status may not capture the true causal effect of interest, since some individuals may not comply with their assignment or may drop out of the program. For the heterogeneous treatment effects of the new variables, I present a transformed potential outcome forest algorithm, a variant of the random forest algorithm that splits the nodes according to a criterion that maximizes the variance of the transformed potential outcomes.
The views in this Working Paper Class are those of the authors, not necessarily of Machine Learning X Doing.
Opoku-Agyemang, Kweku A. (2023). "Super Dummy Variables." Machine Learning X Doing Working Paper Class 6. Machine Learning X Doing.
Copyright © 2023 Machine Learning X Doing Incorporated. All Rights Reserved.
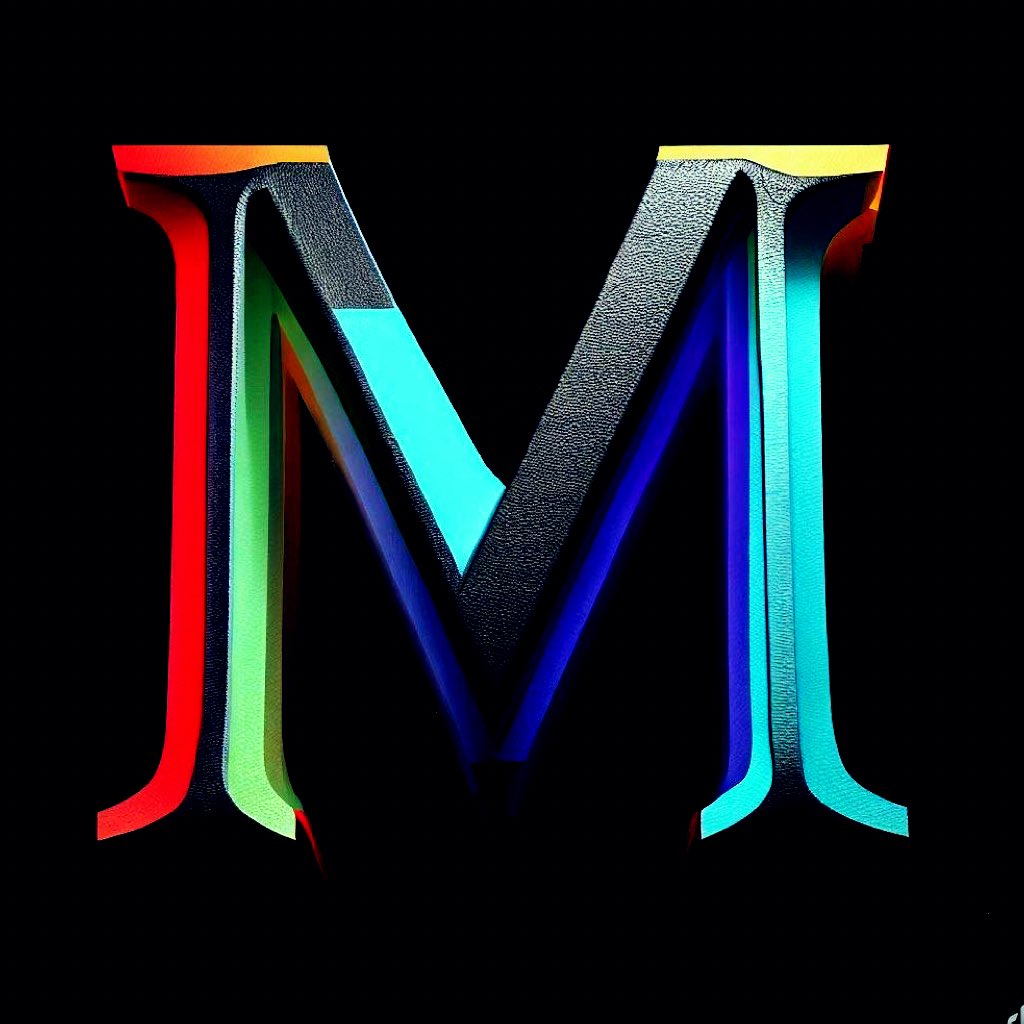